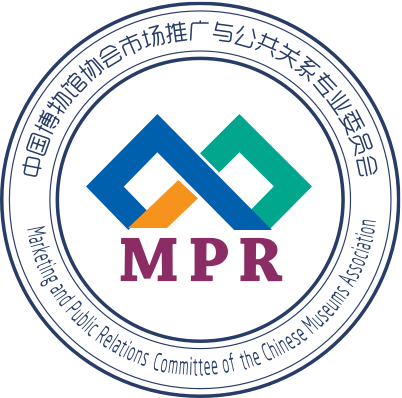
Machine learning predicts interaction time between museum visitors and exhibits
- MPR
- Time:2020-12-16 13:45
In a proof of concept study, education and artificial intelligence researchers demonstrated that machine learning models can be used to predict the interaction time between visitors and specific exhibits in a museum. This discovery opens the door to a number of new jobs aimed at enhancing interaction between users and informal learning tools.
"Education is an important part of most museums' mission statements," said Jonathan Rowe, a research scientist at the center for educational information at North Carolina State University (CEI), co-author of the study. "The amount of time people interact with exhibits can be a measure of engagement and help us assess the quality of the learning experience in a museum environment. After all, it's not the same as in school. Visitors can't take the exam. "
"If we can determine how long people will spend on an exhibit, or how long it will take for people to lose interest in an exhibit, we can use this information to curate and arrange the exhibition reasonably, conform to the rules of user behavior, and maintain audience participation," lead author Andrew Emerson, PhD student at North Carolina State University Emerson).
To determine how machine learning programs predict user interaction time, the researchers closely monitored the interaction between 85 museum visitors and an environmental science interactive exhibit. Specifically, the researchers collected data on participants' facial expressions, posture, location of viewing display screen and position of touching screen.
These data are then input into five different machine learning models to determine which combination of data and model can produce the most accurate prediction.
“We found that a machine learning method called 'random forests' works very well, even using only pose and facial expression data to get good results." Emerson said.
The researchers also found that the longer people interact with exhibits, the better the model works, because it provides more data for the model. For example, the prediction made by the model in a few minutes is more accurate than that made after 30 seconds. According to this study, the interaction time between users and exhibits is as long as 12 minutes.
"We're excited about this because it paves the way for new ways for audiences to learn in museums," Luo said. "We hope to eventually use technology to improve learning efficiency and make learning more attractive."
This paper, early prediction of visitor engagement in science museums with multimodal learning analytics, was published at the 22nd ACM multimodal interaction (ICMI'20) online from October 25 to 29. The paper is written by Nathan Henderson, PhD student of North Carolina State University, wookhee min and Seung Lee, CEI research scientists of North Carolina State University, and James Minogue, associate professor of teacher education and learning science at North Carolina State University Minogue and James Lester, Professor of computer science at the University of North Carolina and director of CEI.
This work was funded by the National Science Foundation (NSF) No. 1713545.
The following is a summary of the study
Early prediction of science museum audience participation using multimodal learning analysis
Abstract: in informal learning environments such as museums and science centers, establishing audience participation model is an important challenge. It has great potential to design a prediction model of audience participation and accurately predict the significant characteristics of audience behavior, such as residence time, in the rational design of learning environment and audience analysis in museums and science centers. This paper introduces a multimodal early prediction method, which can simulate the audience's participation in Environmental Science interactive exhibits. In the process of interaction between visitors and environmental science exhibits, we collected multi-modal sensor data, including eye focus, facial expression, posture and interactive log data, and used these data to summarize the prediction model of visitors' stay time. In this paper, several machine learning techniques (random forest, support vector machine, Lasso regression, gradient lifting tree and multi-layer perceptron) are studied. Based on the data of 85 museum visitors, the multimodal prediction model of audience participation is concluded. The results of a series of ablation experiments show that the accuracy of the model can be improved by incorporating additional modes into the prediction model of audience participation. In addition, with the passage of time, the prediction performance of the model display has improved, which shows that as the model obtains more and more data from the interaction between the audience and the environmental science exhibits, it can make more and more accurate prediction of the audience stay time. These findings highlight the positive role of multimodal data in modeling audience participation in museum exhibits.
2020-11-18
Editor: Pumpkin
Recommended Content
-
From offline to online, how can digital technology reshape museums?
2020-12-16 13:38 -
Scientific and technological innovation promotes cultural confidence, and the 2020 collaborative platform Conference on the industrialization and application of cultural relics protection equipment was held
2020-12-16 13:59 -
The platform construction project of Digital Museum of China's lost overseas Artworks (not displayed) will be launched soon
2020-12-16 14:03 -
Announcement of the final evaluation results of the first China Exhibition Art and exhibition technology innovation competition
2020-12-16 14:44
News
-
Announcement of the final evaluation results of the first China Exhibition Art and exhibition technology innovation competition
-
"The oldest smiling face" in the world at the National Museum of Ukraine
-
Handling | There are more options for IP development
-
Handling | development of cultural and Museum IP: building an effective connection with the audience based on people
-
Carrying, cultural and Museum IP helps cultural relics "live"
-
The platform construction project of Digital Museum of China's lost overseas Artworks (not displayed) will be launched soon
-
Scientific and technological innovation promotes cultural confidence, and the 2020 collaborative platform Conference on the industrialization and application of cultural relics protection equipment was held
-
Machine learning predicts interaction time between museum visitors and exhibits
-
From offline to online, how can digital technology reshape museums?
-
Cultural and natural heritage day | intangible cultural heritage "in the clouds" and "show" in the studio